Highlights of the 39th International Symposium on Forecasting: what you might have missed
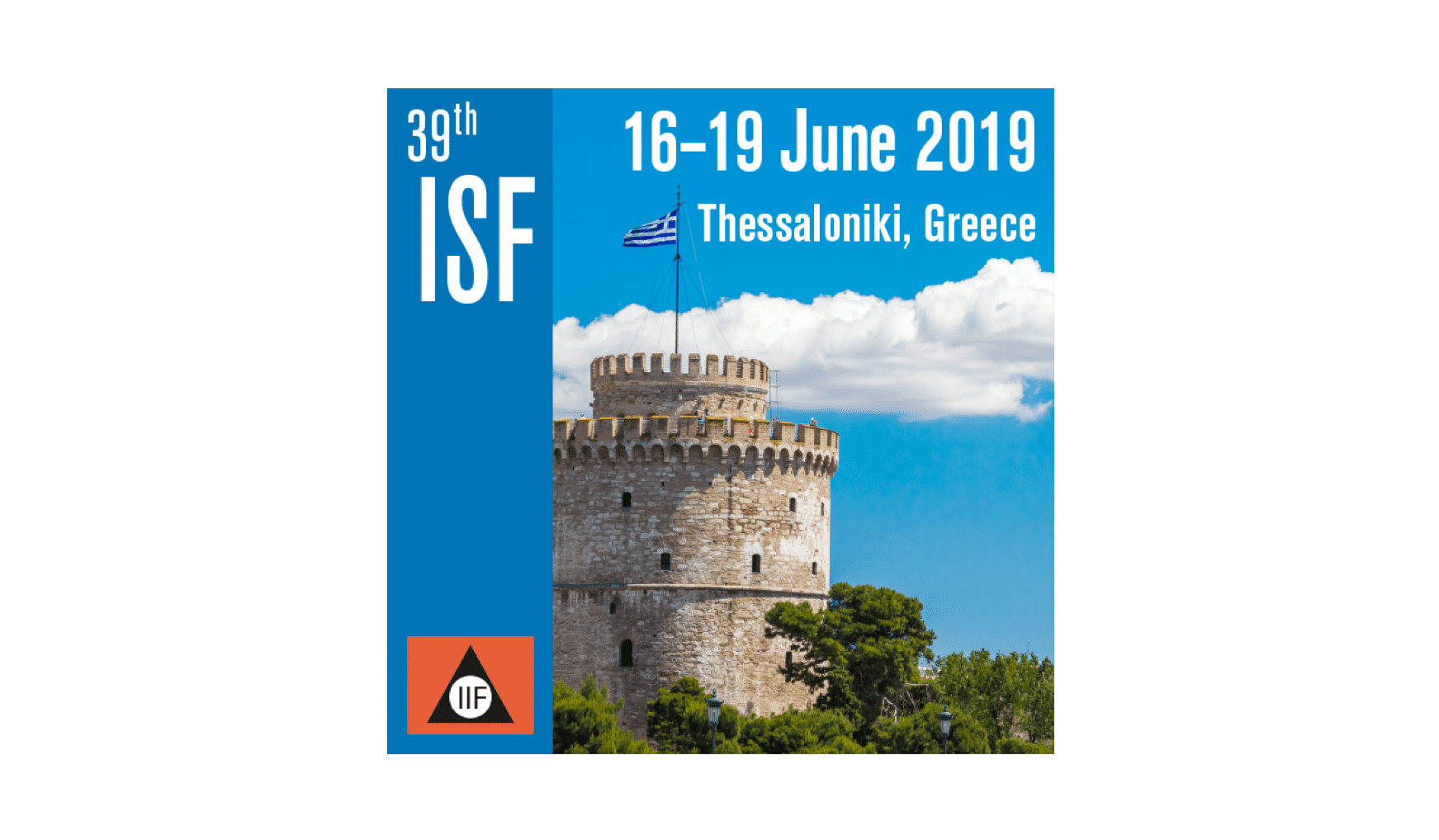
Thessaloniki, Greece – June 2019
The International Symposium of Forecasting is the greatest forecasting conference, attracting the world’s leading forecasting researchers, practitioners, and students. 400 people coming from 35 different countries attended the event. They represented a large industry spectrum (Banking, Government agencies, Utilities, Supply chain forecasting, Airlines, Pharmaceuticals, Forecasting software,…). This event connects indeed academics and industry together, and is the right place to share knowledge and feed each other through keynotes and workshops.
We report below the main themes and insights from this event, on both business and technical sides.
Key business insights
Sales vs Demand
In contrast to weather forecast, historic times series is strongly impacted by the forecasting process. There is a significant difference between sales and demand. A sales time series may contain values that do not reflect demand (zero sales, outliers, substitution effects…).
For example, Renault-Nissan presented how they realize short-run operational forecasts. In their case, predicting the sales at the “mix level” is appropriate : i.e. the combination of technical features defining a vehicle (color, engine, energy, gear…). Autoregressive linear forecasting tend to decrease the bullwhip effect. But when relying only on historical demand data and inventory data, hierarchical and grouped time series perform best for mix forecasts.
Demand influencing factors are sometimes not taken into account : no information about promotions, external factors not modeled (new store, weather), unreasonable information (negative prices, mis-scans of products…). Causal forecasting will help to include these demand influencing factors : you also need to forecast these predictors. A quantile forecast is also very important in the Retail industry, the uncertainty of the forecast will be reflected with a safety stock. However improved forecast accuracy will not always directly translate into better logistical orders. The « best » point forecast depends on the error or accuracy measure : it can be the average, the median…
Impacts on Pricing
There are 3 situations with changing price : new items, investments and inventory management. At Walmart, the pricing change for inventory management is currently challenging. Indeed, the clearance of items must lead to no empty shelves, maximizing revenues and getting rid of sell-through inventory. During clearance cadence, the price will drop several times while the sell-through rate will increase up to 100%. The price elasticity is a measure to show the responsiveness, of the quantity demanded of a good or service to a change in its price when nothing but the price changes. The price elasticity is not linear and price changes are not synchronized from one store to another. Items are cleared only once, so there is no history of clearance. Other confounding factors like store item level placement, cannibalization, rare events, variation in weather make the modeling challenging. The best approach for Walmart is the decomposition with a base forecast and adding the elasticity as this is reasonable to explain for forecasters.
Spare parts & remanufacturing
The concept of circular economy is to keep resources in the system. Re-manufacturers have to deal with extra uncertainties. Unlike demand, the stream of returned items is not an independent random variable, it correlates with past sales. Thus, univariate approaches will underperform. There are varying options of available information regarding the relationship between returned items and past sales : assume that every sale is accompanied by a return, relate returns to past sales or track returns to past sales. For the last option, serialized information allows us to correlate and track return to past sales and update the true number of items sold in each previous periods, not yet returned. Serialization improves forecast accuracy for both slow and fast items. Moving from returns to net demand forecasts would be a next step.
Spare parts are characterized with intermittent demand which is a challenging task to predict and stock-outs will imply tremendous costs. Different approaches have been proposed in the literature to address these issues, a new approach presented was a Bayesian method based on the Compound Poisson distribution to model spare parts demand : it assumes that the parameters of the lead-time demand distribution are random and characterized with a prior distribution. This approach can be compared to parametric methods as benchmark (Croston, SBA, ADIDA…).
Integrating those forecasts of spare parts to the net demand forecast would be a next step for research.
The Supply chain of tomorrow
The rate of technological change is accelerating drastically : autonomous vehicles, drone deliveries, 5G, IoT… Geo-political turbulences have huge impacts on supply chains. The belt and road initiative (BRI) created a global infrastructure network and will keep improving the set of sea and rail links between China and Europe. People prefer access over ownership, the business model of tomorrow is Product as a service. Warehousing providers are also adapting to the same model, products must be closer to the consumption point. The supply chain of the future will be characterized by :
- distributed manufacturing
- circular, local and rapid supply chains
- modular products, more personalized and thereby closer to customer demand
- shared economy
There is a shift from physical to digital inventory, produced on-demand worldwide. Inventory levels will decline with fewer finished goods in stock. We will see an increase in returns, re-use and recycling. Demand has changed fundamentally.
Despite advances in technology that ease information sharing, point of sales (POS) data are not widely used for demand forecasting. The investigation of a two echelon supply chain where the manufacturer does not have access to the retailer’s inventory and the demand is highly variable due to promotions, shows that the POS data improve the accuracy of demand forecasting.
Another challenge is to forecast demand for new products. Pre-release buzz for new products can help the forecast of these products : Google Trends provide such information. It is well understood that homogeneous products follow similar patterns. Building pre-release buzz patterns help the prediction of products’ success. This can be done with competitors’ products too to gain insights about the market. Then, the idea is to adjust advertising strategies and operational decisions.
Finally, the role of the forecaster needs to be redefined. The Forecast Value Added represents the change in a forecasting performance metric (for example MAPE) that can be attributed to a particular step or participants in the forecasting process. Small adjustments don’t improve forecast accuracy, a Morlidge’s research has shown that 52% of companies studied were less accurate than a naïve no-change forecast. Identifying and eliminating these non-value adding activities with a Forecast Value Added analysis will help forecasters to focus on items with high value and hard to predict.
Key technical insights
Probabilistic forecasting
Amazon AI has released GluonTS, a time series probabilistic forecasting framework. It integrates built-in models such as DeepAR and also provides datasets (M3, M4 competitions, sales, electricity datasets). The concern of delivering the prediction interval associated to the point forecasts is of prime importance, especially for avoiding stock outs or overstocks. Amazon AI states that GluonTS can perform well with 300-400 time series. From our point of view, GluonTS is quite easy to use . We might present it and its key features in a further blog post, so stay tuned.
Combining Statistical and ML/Deep Learning-based forecasting methods
The conference was also the opportunity to demonstrate the potential of combining machine learning and statistical models.
Amazon AI presented a probabilistic forecasting approach combining state space models and deep learning. In a nutshell, it combines a per-time-series linear state space model with a jointly-learned recurrent neural network, allowing to retain state space model properties like efficiency and interpretability, while giving the ability to learn complex patterns from large collection of data with deep learning algorithms.
On deep learning methods, Microsoft presented their submission to the M4 competition, based on Google Wavenet (dilated causal convolutional layer) and concrete dropout (Gal, Yarin et al. Concrete dropout. Advances in neural information processing, 2017), a regularization technique that allows dropout rates to be learned during the training phase. One interesting benefit of concrete dropout is that a prediction interval can be obtained at prediction time.
The talk by SAS aimed to demonstrate that machine learning and traditional approaches perform poorly alone but better when combined, especially when the data is sparse and noisy, or when trying to forecast on short and long term time series with high variability.
This point of view is shared by Quantmetry, as our lead of Time Series Practice, gave a talk on statistical and machine learning methods combination for improved energy consumption forecasting performance. Based on a competition organized by Quantmetry and EDF, the French energy supplier, we shared best practices on time series modeling with machine learning methods, demonstrating that data cleaning, feature engineering and open data feature augmentation, combined to statistical and machine learning mixed models are key to performance improvement.
Hierarchical forecasting
In many situations, products can be ordered hierarchically. Forecasts are needed at all levels, but how can we reconcile the forecasts so that all the levels are consistent and sum up?
A talk given by a Data Scientist at Renault-Nissan showed that HTS (Hierarchical Time Series) approach outperformed classical approaches to forecast sales more accurately at the mix level.
The methodology used involved LSTM networks to efficiently model the long-term dependencies in this multivariate time-series problem, and a combination of hierarchical forecast methods suited to disaggregated forecasts on product hierarchy.
Devon Barrow (University of Birmingham), presented interesting work on temporal aggregation and the improvement/loss in forecast accuracy with regards to the forecast horizon. Specifically, the MAPA (multiple aggregation prediction algorithm) and THieF (temporal hierarchies) methods proved that they perform better, especially for longer forecast horizons . Devon proposed a methodology to dynamically change the temporal aggregation range depending on the forecast horizon. This research work still needs further investigation, but the first results give interesting insights.
M4 Competition
One of the most awaited moment of the conference was the talk given by Slawek Smyl from Uber technologies, presenting the M4 competition winning approach.
This sophisticated method is based on a combination of ETS (exponential smoothing) equations and RNN (shallow networks), with the help of an ensemble of specialist models: at train time, several models see a subset of the time series on which they perform best. This is repeated at each epoch, and shows significant improvement in the results.
The organisers were very enthusiastic on the next competition, the M5, and announced that it will focus more on real-world data.
During a keynote session, Spyros Makridakis was a bit skeptical on deep learning/machine learning methods used alone for forecasting, as pure machine learning methods performed poorly during the M4 competition. No doubt that the future M5 competition will push further the knowledge on deep learning for forecasting, with all eyes on these methods.
The Future of Forecasting
Despite giving interesting insights on how to combine statistical and machine learning methods to create better forecasts, the biggest criticism made to the M4 is that the time series came by themselves, without any external data or additional information.
As a response to the community, the future M5 competition will be more focused on:
- Intermittent demand
- Realistic/real-life forecasting
- Hierarchies
- New products
- Messy data
- And explanatory/exogenous variables integration
More concerns on estimating predictions intervals and communicating on the uncertainty of the forecasts will be of interests in the next couple years. Unifying the forecasting field and defining the role of Full-time forecaster will also be major stakes.
✍Written by Charlotte Ledoux, Senior Data Consultant, and Guillaume Hochard, Time Series Practice Lead, @Quantmetry